About
Ride-hailing systems have increased congestion in many cities. For instance, a recent study has shown that, between 2010 and 2016, weekday vehicle hours of delay increased by 62% compared to 22% in a counterfactual 2016 scenario without ride hailing. Large-scale ride-sharing can change the equation. recently, researchers at MIT have shown that 98% of the riders using NYC taxi and limousine services in NY city can be served with 3,000 vehicles and an average wait of 3.8 minutes (NYC has currently about 12,000 taxis). This project aims at designing large-scale ride-sharing systems that serves all the rides with short waiting times and small deviation compared to the shortest paths of the riders. The underlying technology combines optimization, machine learning, and model-predictive control.
Large-Scale Ride-Sharing Systems
Impact
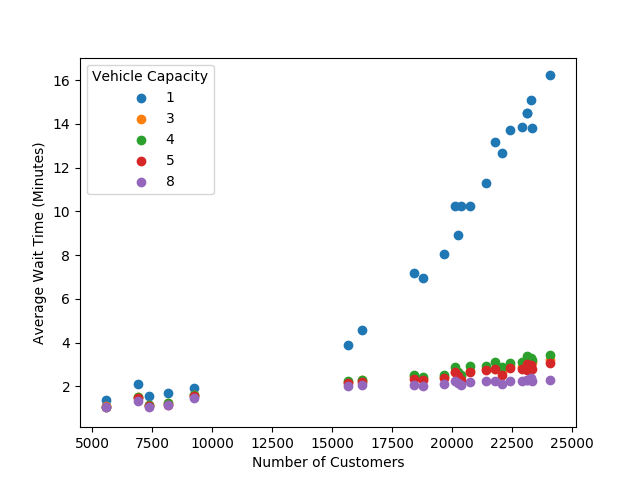
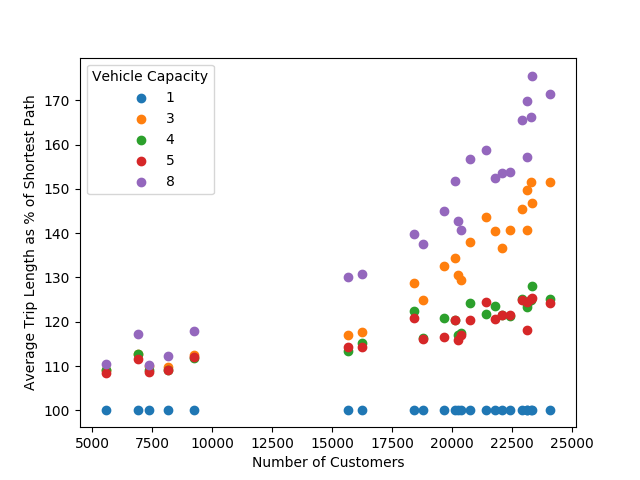
Recent results using a dedicated optimization algorithm has shown that all riders can be served with 2,000 vehicles, an average wait time of 2.2 minutes, and an average deviation of 0.62 minute compared to a direct trip. The figures above depict some of these results and report the average waiting times and average vehicle occupancy for varying numbers of customers and fleet sizes during peak hours. Interestingly, the average occupancy is around 1.3 as soon as there are more than 2,000 vehicles in the fleet. This indicates that ride-sharing does not typically lead to overcrowded vehicles.
Researchers
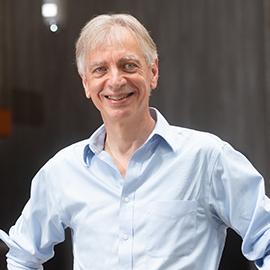