About
Parking occupies a significant portion of our cities: There are at least 800 million parking spaces in the United States alone and 14% of Los Angeles County is devoted to parking. Parking also contributes to congestion, as the average share of cruising to find a parking spot is 30% in the United States. The community-based trip-sharing project aims at minimizing the number of cars on the roads and pressure on parking.
Car-pooling has long been proposed as a potential solution for reducing peak-hour congestion and parking pressure. Its adoption however is poor in general as 76.4% of American commuters chooses to drive alone. In prior studies, researchers identified the difficulty in finding people with similar location and schedule as the main reason for not car-pooling. There is thus a unique opportunity to build a matching platform based on artificial intelligence and operations research for boosting adoption of car-pooling.
Community-based trip sharing is an embodiment of such a platform: Its key idea is to organize the pooling around commuting communities, exploiting spatial and temporal locality, i.e., the knowledge of when employees arrive on a (corporate or university) campus in the morning and leave in the evening riders. It also guarantees a “ride back” in the evening, probably the most critical factor for adoption. This contrasts with the car-pooling platform Scoop which only provides weak guarantees for “ride back”, with monthly limits on how much auxiliary services can be used when a ride back is not available.
Impact
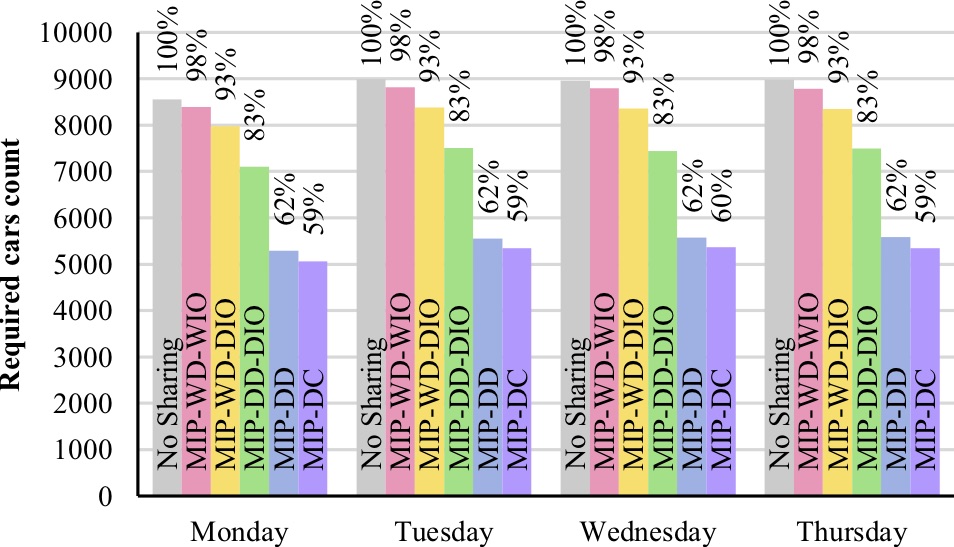
It would be ideal to have the same riders commute together in the morning and the evening, as well as every day of the week. Unfortunately, one of the results of this study is the recognition that riders and drivers must be matched dynamically, every day and every morning and afternoon: It is only when riders are matched dynamically that significant car-pooling occurs. This important realization, which explains the poor adoption in existing car-pooling programs, is illustrated on the city of Ann Arbor. The “No Sharing” column represent the number of cars with no car-pooling. The remaining columns impose progressively fewer constraints on the matching. In particular, column WD-WIO requires that the same routes and drivers are used every day and that the same riders commute together in every morning and evening. As can be seen, these constraints are too stringent: Car-pooling under these conditions reduces the number of cars on the road by 2% only. In contrast, DD only requires that the drivers are the same in the morning and in the evening in order to satisfy the “ride back” constraint: It saves the number of cars by 45% for the entire region and by more than 60% within the city limit. The platform thus needs to match drivers and riders every morning and every afternoon in real-time. Moreover, it is desirable to adjust the afternoon dynamically as riders update their schedules. Optimization algorithm based on route generation are capable to meet these requirements, primarily because they exploit spatial and temporal locality.
Researchers
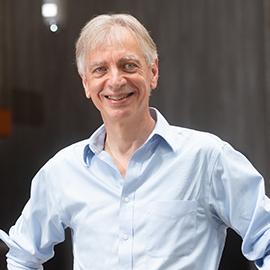
Related Publications
2021
The Benefits of Autonomous Vehicles for Community-Based Trip Sharing. Hafiz Hasan and Pascal Van Hentenryck. Transportation Research Part C, 124, March 2021.
Commuting with Autonomous Vehicles: A Branch and Cut Algorithm with Redundant Modeling, Mohd. Hafiz Hasan and Pascal Van Hentenryck. ArXiv:2101.01072.
2020
The Flexible and Real-Time Commute Trip Sharing Problems. Mohd. Hafiz Hasan and Pascal Van Hentenryck. Constraints, 5, 160-179, 2020.
The Commute Trip Sharing Problem. M.H. Hasan, Pascal Van Hentenryck, and Antoine Legrain. Transportation Science, 54(6): 1640-1675, 2020.
2019
On-Demand Mobility Systems, Pascal Van Hentenryck, ISE Magazine, Fall 2019.
2018
Community-Based Trip Sharing for Urban Commuting. Hafiz Hasan, Pascal Van Hentenryck, Ceren Budak, Joyce Chen, and Chhavi Chaudhry. In the Proceedings of The Thirty-Second AAAI Conference on Artificial Intelligence (AAAI-18), New Orleans, LO. February 2–7, 2018.